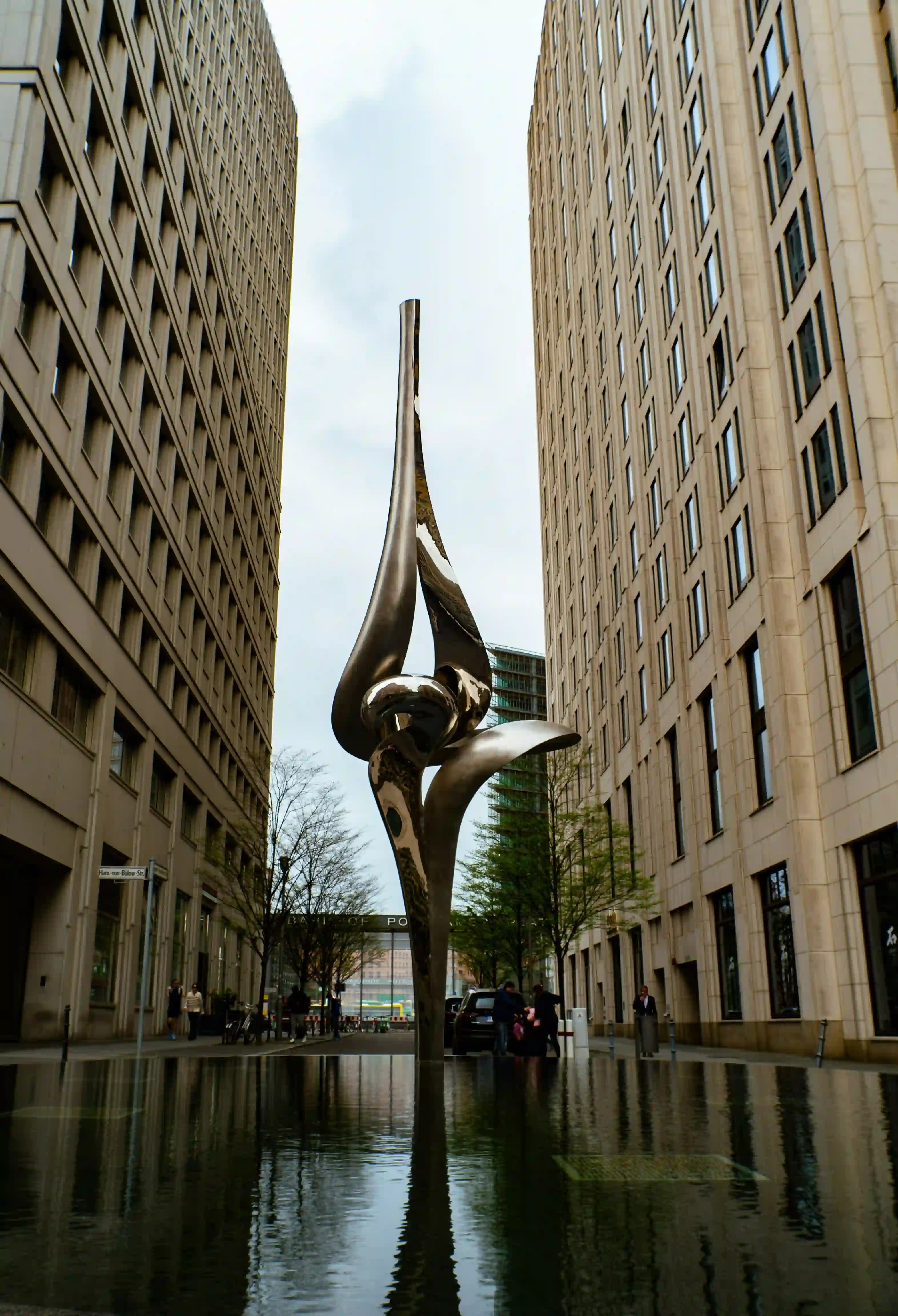
AI in Business: What Leaders Need to Know About the Future of Work
Advertisement
AI Isn't Coming, It's Already Here
Let's be honest: artificial intelligence isn't some distant future concept anymore. Every LinkedIn post, news headline, and boardroom discussion seems to revolve around it. And if you're in business, whether you're an executive, manager, or entrepreneur, you've probably wodered: What does this actually mean for me?
The AI Jargon You Can't Ignore (But Might Not Fully Understand)
First things first, let's cut through the "acronym soup." You've heard terms like AGI (Artificial General Intelligence), LLMs (Large Language Models), and generative AI thrown around. Here’s the thing: AGI, the sci-fi dream (or nightmare) of machines surpassing human intelligence, isn’t what we should be losing sleep over right now. The real game-changer? Narrow AI.
Narrow AI is already embedded in your daily life, think facial recognition on your phone or Siri transcribing your voice notes. But what’s shifted recently is how these systems have evolved from just predicting outcomes (like forecasting sales) to creating content, text, images, even music, with tools like ChatGPT. That’s the "generative" part of AI, and it’s rewriting the rules for everything from marketing to customer service.
The Workplace Revolution No One’s Fully Prepared For
Here’s a staggering stat: Consulting firms estimate that 40% of all working hours could be transformed by AI. Not eliminated, transformed. Take customer service roles: research suggests a third of tasks could be automated, another third augmented by AI, and the rest untouched. But here’s the twist: new jobs are emerging too, like "prompt engineers" (people who craft precise instructions for AI systems) or roles focused on monitoring AI outputs for quality and bias.
The speed boost is undeniable. Morgan Stanley’s GPT-4-powered chatbot, for example, condenses tasks that once took financial advisors 30 minutes into three seconds. But with that efficiency comes big questions: How do we ensure accuracy? Who’s accountable when AI gets it wrong? And most importantly, how do we adapt our teams and workflows?
The Elephant in the Rooom: Ethics and Fear
Let’s address the unease head-on. Yes, AI hallucinates (makes up facts). Yes, it can amplify biases hidden in its training data (try generating an image of "schoolgirls" and see what pops up). And yes, disinformation at scale is a terrifying prospect, especially with elections looming globally.
The solution isn’t to panic or freeze. It’s to engage. Companies diving into AI need two things urgently: (1) clean, organized data (many legacy systems are a mess), and (2) ethical guardrails. Regulation is lagging, except in places like the EU and China, but businesses can’t wait for policies to catch up. Proactive measures, like retraining models on internal data to reduce bias or setting strict usage policies, are already separating leaders from laggards.
A Human-Centric Approach to the AI Era
The biggest misconception? That AI will replace humans outright. The reality is more nuanced: hybrid roles blending human judgment with AI capabilities are becoming the norm. Take hiring, while AI interviews can feel impersonal (and sometimes flawed), they also remove human inconsistencies like mood-based biaases post-lunch.
The bottom line: Whether you're excited or apprehensive about AI, one thing’s certain, ignoring it isn’t an option. The businesses thriving tomorrow are those experimenting today while keeping ethics and adaptability at the core.
The AI Landscape in Business: Understanding the Basics
Artificial Intelligence has evolved from a niche technology to a business imperative almost overnight. While the buzz around AI is impossible to ignore, many professionals still struggle with fundamental questions: What exactly are we talking about when we discuss AI in business contexts? And more importantly, how does this translate to real-world applications?
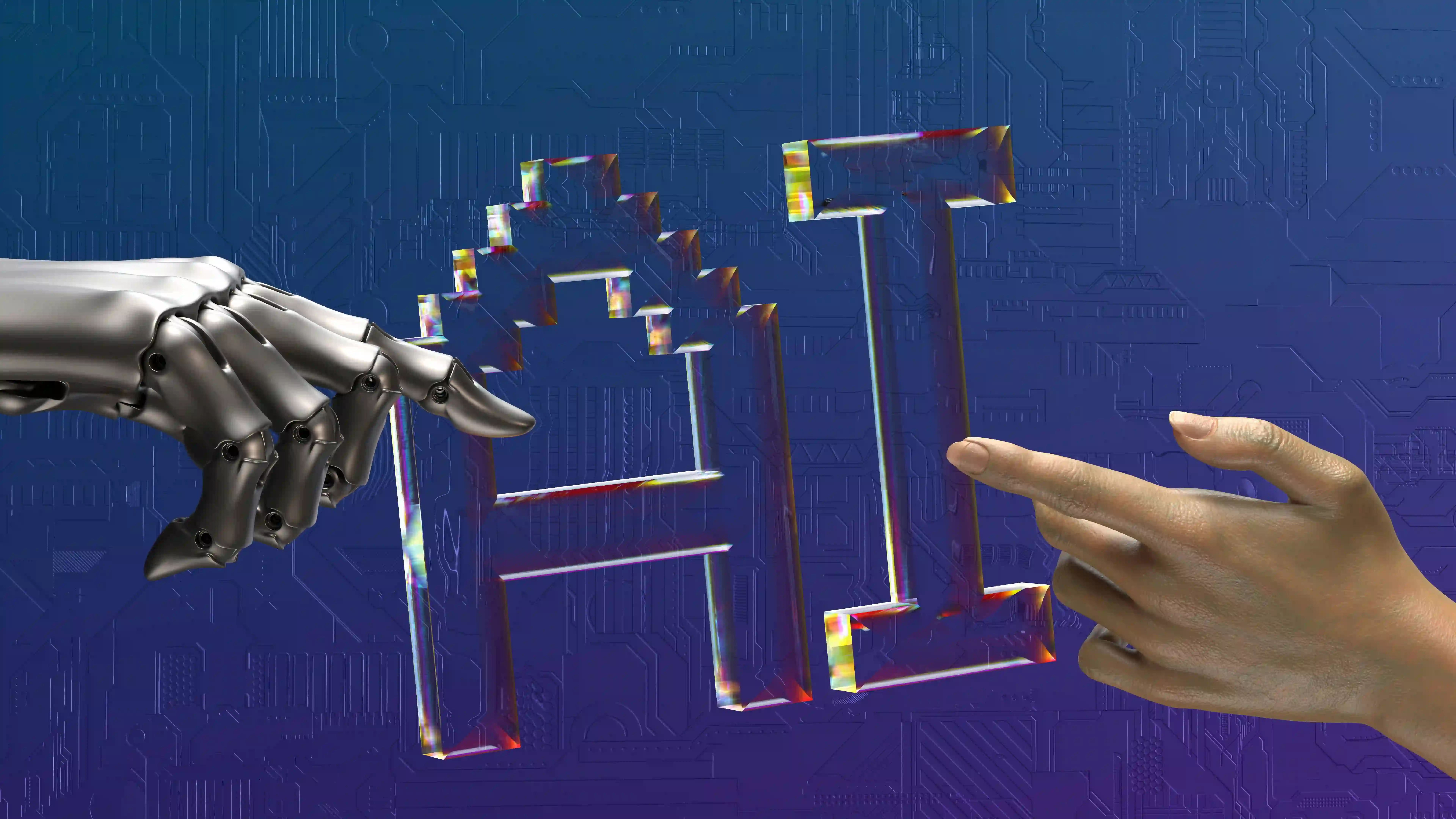
The Terminology Maze
Let's start by untangling some key terms that often get thrown around interchangeably:
- Narrow AI: Systems designed for specific tasks (think facial recognition or spam filters)
- Generative AI: Tools that create original content - text, images, code
- LLMs (Large Language Models): The technology powering tools like ChatGPT that understand and generate human-like text
AI's Impact on the Workplace: Beyond the Hype
The real transformation is happening in how these technologies are reshaping work itself. Consider these emerging patterns:
Job Category | Impact Level | Example Changes |
---|---|---|
Customer Service | High Transformation | AI handling routine queries, humans focusing on complex cases |
Financial Services | Moderate Augmentation | Analysts using AI to process data faster while maintaining decision authority |
Creative Fields | Emerging Disruption | Designers collaborating with image generation tools for rapid prototyping |
The Rise of Hybrid Roles
What's particularly fascinating is how job descriptions are evolving. We're seeing entirely new positions emerge at the intersection of human expertise and AI capabilities:
- Prompt Engineers: Professionals who specialize in crafting effective inputs for generative AI systems.
- AI Trainers: Experts who fine-tune models using company-specific data and knowledge.
- Ethics Specialists: Teams focused on ensuring responsible AI deployment within organizations.
The most successful implementations we're seeing don't replace humans but rather create collaborative workflows where each plays to their strengths. A financial advisor might use an AI assistant to instantly surface relevant regulations, but still apply human judgment in interpreting them for clients.
The Implementation Challenge: Bridging Theory and Practice
While the potential is enormous, companies face very real hurdles when moving from experimentation to production:
A common pattern emerges when businesses attempt adoption - they often don't know where to start. The most frequent requests consultants receive are surprisingly vague: "We need AI" or "Make us an AI company." This reflects both enthusiasm and uncertainty about how to proceed strategically.
The Data Foundation Problem
The single biggest obstacle isn't the AI technology itself but what feeds it. Many established companies discover their data infrastructure resembles an archeological dig - layers upon layers of systems with inconsistent formats, missing metadata, and unclear ownership.
A sobering truth: The quality of your outputs will never exceed the quality of your inputs. Before investing in flashy AI solutions, most organizations need to invest in basic data hygiene and governance.
The Human Factor: Adoption Beyond Technology
The technical challenges, while significant, may actually be easier to solve than the human ones. Employees across organizations typically fall into three camps when facing AI adoption:
- The Enthusiasts (15-20%): "How can I use this today?" Often experiment without waitng for formal approval.
- The Cautious Majority (60-70%): "Will this replace me?" Need reassurance about changing rather than disappearing roles.
- The Resisters (10-20%): "This will never work here." May actively undermine adoption efforts if not engaged properly.
- LLMs (Large Language Models): Trained on trillions of words to understand and generate human-like text
- Foundational Models: The underlying architectures powering today's most advanced AI systems
- Neural Networks: Complex systems modeled after the human brain's structure
- Customer service: Morgan Stanley reduced some financial advisor tasks from 30 minutes to 3 seconds using approved internal chatbots
- Knowledge work: Legal document review that once took days now happens in minutes with higher accuracy
- Talent acquisition: While controversial, AI screening tools process thousands of applications consistently where humans show bias
- Tame unstructured data: Decades of documents in incompatible formats create integration nightmares
- Establish governance: Without clear data policies, even the best models produce unreliable outputs
- Cultivate new roles: Data curators and prompt engineers are becoming as crucial as traditional IT staff
- Candidates often glorify AI assessments as more objective than human interviews
- The experience proves emotionally draining without human feedback cues
- Candidates adapt behavior in unnatural ways when interacting with machines versus people
- Toxicity amplifiication: "Ask for an image of 'schoolboys' versus 'schoolgirls' and you'll see disturbing differences reflecting internet biases" one expert warned
- Synthetic disinformation: The coming election cycles will test democracies' resilience against machine-generated propaganda
- Cognitive labor shifts: "We're outsourcing not just physical tasks but increasingly our thinking processes" notes an organizational psychologist
- Socioeconomic divides: Crisis of authenticity:
Successful implementations address all three groups through tailored communication and training programs that go far beyond technical how-to's into mindset shifts and new ways of working.
The AI Revolution in Business: What You Need to Know
Artificial intelligence isn't just changing business, it's rewriting the rules entirely. While headlines focus on futuristic scenarios, the real transformation is happening in mundane spreadsheets, customer service calls, and hiring processes. The gap between AI's potential and most organizations' understanding remains vast, but those who bridge it firt will gain significant advantages.
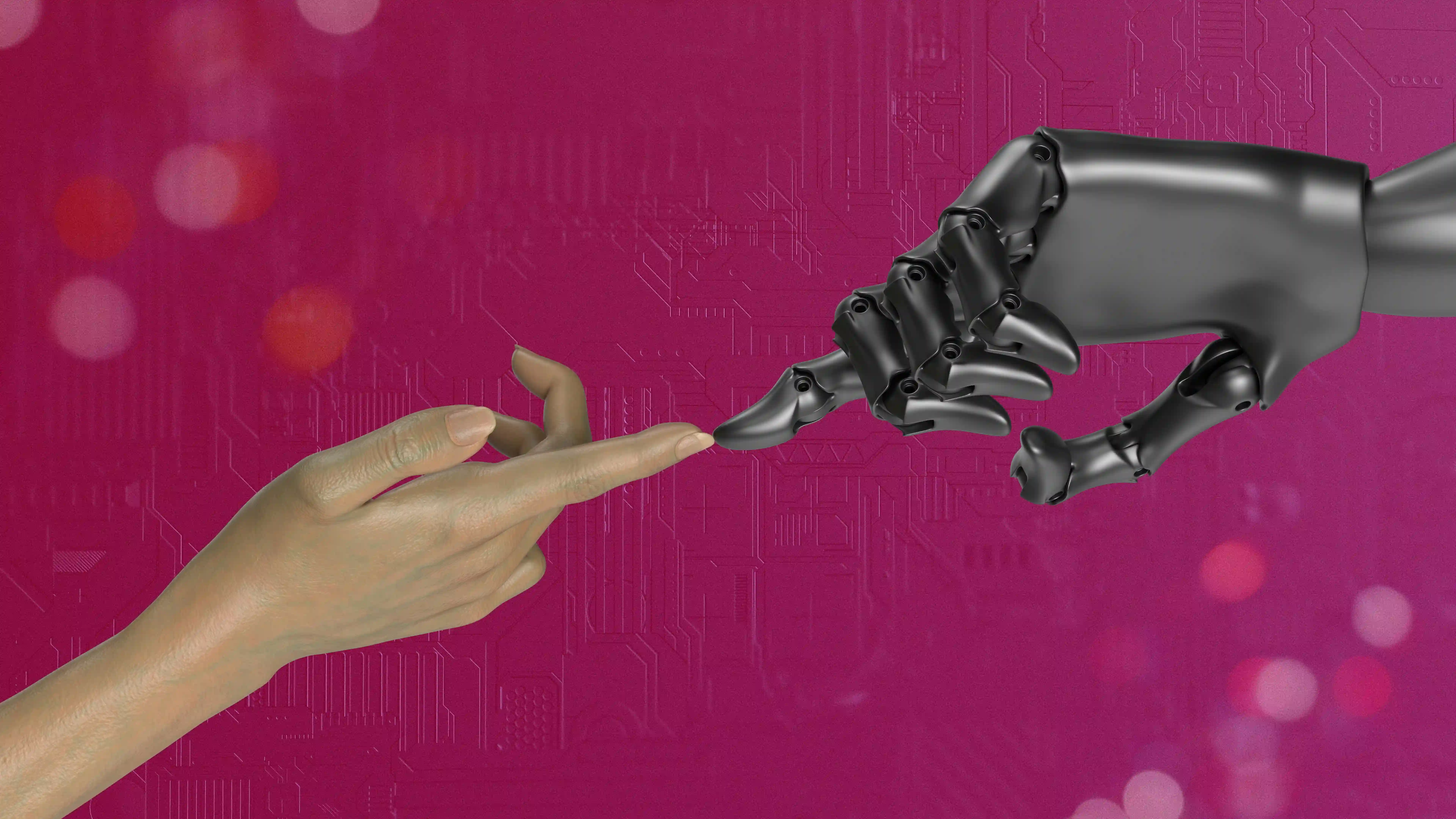
Understanding the AI Landscape
Let's cut through the jargon soup. Narrow AI, systems designed for specific tasks, has been quietly revolutionizing industries for years. phone's facial recognition? That's narrow AI. The seismic shift came when we moved from predictive AI to generative AI that creates original content.
The technology behind this revolution includes:
The Workplace Transformation Already Underway
Consulting firms estimate that 40% of all working hours could be transformed by AI. This isn't about replacing humans but fundamentally changing how work gets done:
The most successful implementations treat AI as a "copilot" rather than replacement.
The Data Dilemma No One Talks About
A dirty secret in enterprise AI adoption? Most companies' data is a mess. Before implementing sophisticated solutions, organizations must:
"We've had clients ask us to 'make AI happen,'" one consultant noted, "without realizing their first step should be a complete data audit." This foundational work separates flashy demos from production-ready solutions.
The Human Side of Technological Change
The psychological impact of interacting with intelligent systems reveals fascinating tensions. Research shows people simultaneously feel both dehumanized by and overly trusting of algorithmic decisions, what psychologists call "automation bias." In hiring contexts specifically:
The Ethics Imperative Can't Wait
Sobering realities demand attention now, not when regulations eventually catch up:

These challenges aren't hypothetical, they're emerging daily in HR departments using emotion recognition software (with questionable cultural validity) and marketing teams deployng generative tools trained on copyrighted material. The solution space requires multidisciplinary thinking combining technical, legal, psychological, and business perspectives. < h4 >Where Do We Go From Here? < p >The path forward balances urgency with responsibility: < ul > < li >< b >Demystify through education:< / b > Every professional should develop basic "AI literacy", understanding capabilities without needing coding skills < li >< b >Pressure-test use cases:< / b > Pilot projects reveal operational realities no white paper can predict < li >< b >Invest in augmentation:< / b > Tools should enhance human judgment rather than replace it entirely < li >< b >Demand transparency:< / b > When vendors claim "AI-powered," ask exactly what that means operationally The conversation around AI in the workplace is evolving at a breakneck pace, and businesses must adapt, not just to survive, but to thrive. From generative AI’s creative capabilities to the ethical dilemmas it introduces, one thing is clear: AI is no longer a futuristic concept, it’s here, reshaping industries, roles, and workflows. 1. Understand the Fundamentals: Whether it's distinguishing between narrow AI (task-specific applications) and AGI (theoretical general intelligence), or grasping how large language models (LLMs) function, foundational knowledge is critical. Misconceptions fuel fear, but clarity empowers strategic decision-making. 2. Focus on Augmentation, Not Just Automation: AI isn’t just about replacing jobs, it’s about transforming them. Roles will evolve into hybrid collaborations between humans and machines. For example, customer service teams can leverage AI for instant data retrieval while focusing on empathy and complex problem-solving. 3. Prioritize Data Readiness: Many organizations struggle with fragmented or poor-quality data, a major barrier to AI implementation. Investing in data governance and infrastructure isn’t glamorous, but it’s essential for reliable AI outcomes. 4. Ethical AI Is Non-Negotiable: From bias in hiring algorithms to disinformation risks, the ethical challenges are real. Businesses must proactively address these concerns through transparency, bias mitigation, and adherence to emerging regulations like the EU AI Act. The rise of AI demands a balanced approach: embrace innovation, but anchor it in human-centric values. For professionals, continuous learning, whether through experimenting with tools like ChatGPT or upskilling in prompt engineering, will be key. For organizations, success lies in strategic partnerships, pilot projects, and fostering a culture that views AI as a collaborator rather than a threat. The future of work isn’t about humans versus machines, it’s about harnessing their combined potential to solve problems faster, smarter, and more equitably. The time to engage is now.Final Thoughts: Navigating the AI Revolution in Business
Key Takeaways for Leaders and Professionals
The Path Forward
Advertisement